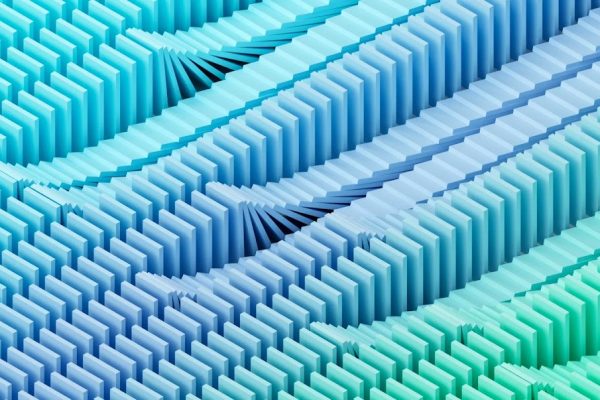
Google DeepMind Introduces the Frontier Safety Framework: A Set of Protocols Designed to Identify & Mitigate Potential Harms Related to Future AI Systems
As AI technology progresses, models may acquire powerful capabilities that could be misused, resulting in significant risks in high-stakes domains such as autonomy, cybersecurity, biosecurity, and machine learning research and development. The key challenge is to ensure that any advancement in AI systems is developed and deployed safely, aligning with human values and societal goals while preventing potential misuse. Google DeepMind introduced the Frontier Safety Framework to address the future risks posed by advanced AI models, particularly the potential for these models to develop capabilities that could cause severe harm.