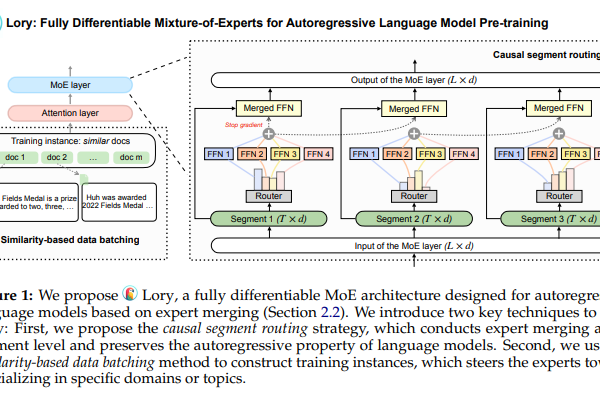
Researchers from Princeton and Meta AI Introduce ‘Lory’: A Fully-Differentiable MoE Model Designed for Autoregressive Language Model Pre-Training
Mixture-of-experts (MoE) architectures use sparse activation to initial the scaling of model sizes while preserving high training and inference efficiency. However, training the router network creates the challenge of optimizing a non-differentiable, discrete objective despite the efficient scaling by MoE models. Recently, an MoE architecture called SMEAR was introduced, which is fully non-differentiable and merges experts gently in the parameter space. SMEAR is very efficient, but its effectiveness is limited to small-scale fine-tuning experiments on downstream classification tasks.